Machine learning (ML) and generative AI have the potential to transform production workflows and the content creation process. But the extent to which they do so will depend on the level of disruption that consumers, writers, talent, and producers have an appetite for.
We do not expect generative AI to entirely replace the creative work done by humans, especially for premium content. Lower-quality or high-churn content—such as social media, email marketing, and basic script writing—will be almost fully automated in the next few years. But human beings will continue to do the heavy lifting at the top end of the spectrum.
Instead, we believe AI should be viewed as a powerful tool that complements the creative process, enhancing productivity and capabilities while a human sits in the driver's seat. It can streamline tasks, offer insights, and assist in aspects of content creation that require a lower degree of creativity, such as summarizing or simple editing. This will enable creators to focus on artistry and innovation.
As a result, we should separate the hype from the near-term promise when exploring what GenAI can do for media and entertainment operations. Below, we lay out specific solutions and practical approaches for implementing the technology.
Where is the creator economy on the AI adoption curve?
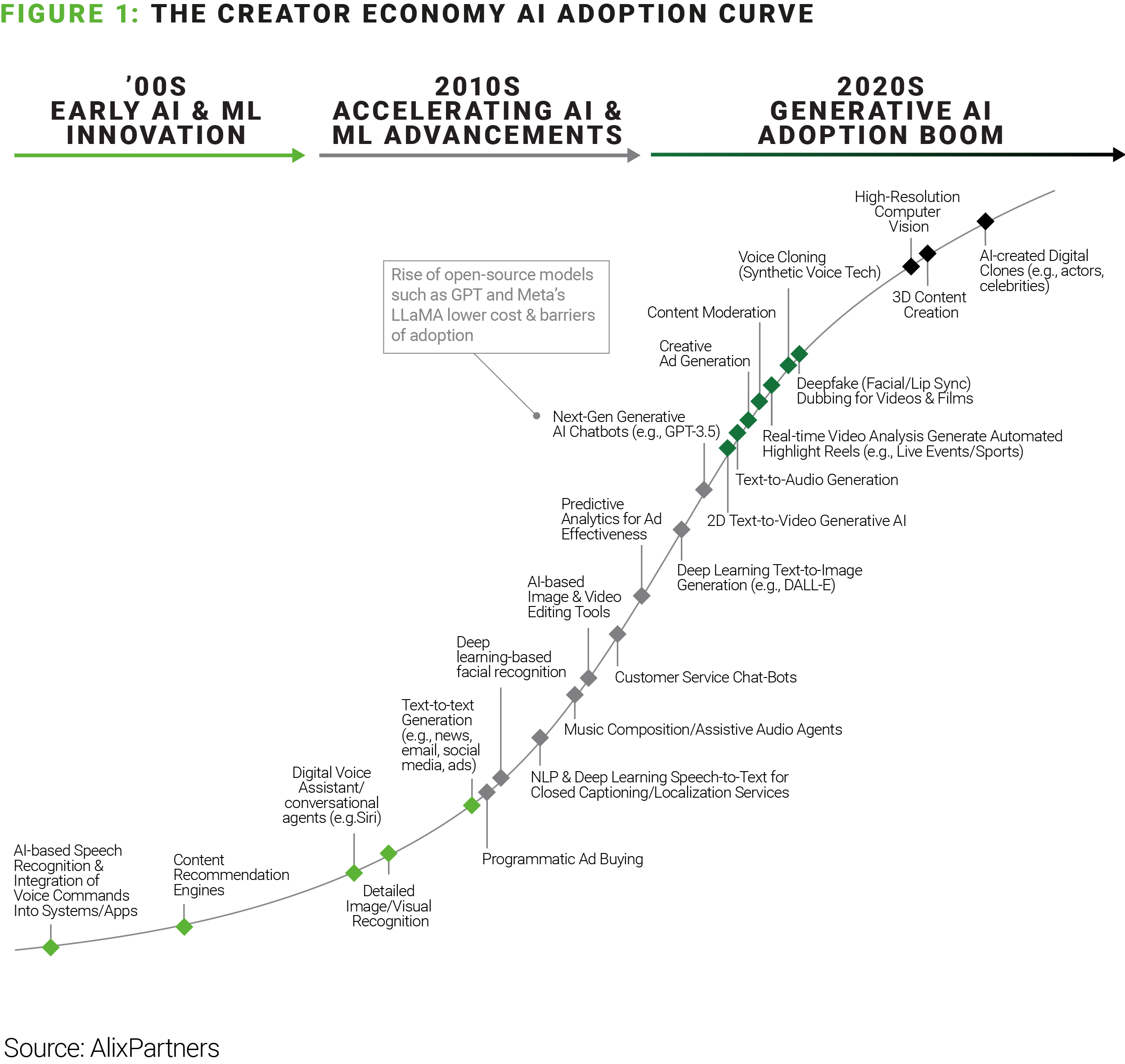
Three examples of rising generative AI use cases that we predict will become more widely adopted by the creator economy in 2024:
- Creative ad generation and modification: GenAI-enabled tools can streamline ad creative processes, enhance ad performance, and adapt content effectively—ultimately improving campaign performance and ROI. Third-party vendor tools can automate simple tasks like designing basic visuals and generating text and headlines, and can also apply deep learning to produce personalized ads across various social channels.
- Post-production use cases such as simple editing or deepfake dubbing for localization and transcription services: Companies like Iyuno—used by Netflix during the postproduction editing process—are leading the innovation charge in the dubbing and localization services space. Deepfake dubbing leverages AI to generate voiceovers that mimic the lip movements and facial emotions of original on-screen actors. This level of synchronization enhances the overall viewing experience and can make localized content appear more natural and less disjointed. Also, early experimental use cases of voice cloning using AI-enabled synthetic voice technology can generate voiceovers that match original on-screen actors.
As a result of domestic production delays from SAG-AFTRA strikes—the implications of which will extend well into 2024—streaming platforms like Netflix, Apple, and Amazon may increasingly turn to international content to fill gaps in their content libraries for the time being. A shift toward international content could be a near-term accelerator of more widespread deepfake dubbing adoption in film and TV. - Real-time video analysis is boosting monetization for content rights owners: WSC Sports is revolutionizing the sports media landscape by harnessing the power of AI to cut, crop, and distribute automatically generated sports highlight clips in real-time—including transforming horizontal game broadcasts into dynamic vertical video for social and mobile apps. The company, which works with hundreds of leagues and broadcasters, provides its partners with better localization for global distribution and greater personalization through curated highlights by player, type of play, and video duration. This not only streamlines back-end processes but enables faster content delivery which, in turn, enhances the fan experience and engagement.
Another example is AI-enabled advanced search for owned IP across audio and video content archives. These tools recognize and label faces, objects, and actions; identify topics, sentiments, and emotions; extract keywords; automatically transcribe speech; and index all captured data for advanced searchability. They also help drive back-end search functions to identify content that can be repurposed across different platforms, maximizing monetization of existing media assets.
Use cases can further extend to user-centric platforms. Tubi, for example, is pioneering a dynamic search feature powered by ChatGPT-4 to assist viewers in navigating its extensive content library of over 200,000 movies and TV episodes. Over time, machine-learning algorithms will yield hyper-personalized recommendations for users, suggesting content that may align with their preferences and moods—giving them less reason to platform hop.
Over the next year, media & entertainment companies must cut through the hype and apply a practical approach that creates measurable value when integrating AI & ML into operations
We all are familiar with the story "The Tortoise and the Hare"—and a speed-to-impact approach for AI implementation is similar. Instead of rushing into AI projects with unrealistic speed and ambition, a more prudent approach is to start small and focus efforts where you have ample structured data. Just as the tortoise took measured steps in the race, AI projects should begin with well-defined and manageable use cases. This approach isn't about being slow or passive; rather, it's about being deliberate and methodical.
Approximately 80% of the initial effort in AI and ML projects is dedicated to data wrangling. Media and entertainment companies vary on the maturity scale in terms of mining, structuring, and leveraging data. These companies must assess and determine their starting point based on data readiness. While they have treasure troves of proprietary data, it is often not in good enough shape for ML and GenAI. By addressing issues like granularity, data provenance, duplication, and accuracy, companies can transform their unstructured data into a foundation for scalable ML and GenAI solutions.
After establishing a structured data backbone, continuous learning through feedback loops is critical. A well-planned and consistent approach to AI implementation increases effectiveness and likelihood of success. It's not about racing to the finish line but about building a sustainable and accurate AI system that can grow over time.
As media businesses pilot new generative AI tools, a challenging next step will be getting humans to do things differently (e.g., accepting and adopting these tools). For example, organizations that utilize AI and ML tools in the marketing function will shift the majority of their staff operations from producing content to more strategic activities like marketing strategy, planning, and market activation (e.g., identifying white space and attacking the market). This transition might also lead to the merging of cross-functional teams. In this evolving landscape, empathy becomes a pivotal factor—caring for the hearts and minds of the people affected will be crucial for operational orientation and effective change management.
Companies should also strike a careful balance between focusing on AI initiatives and pre-existing revenue drivers in the coming year as they navigate how to make AI a profitable venture.
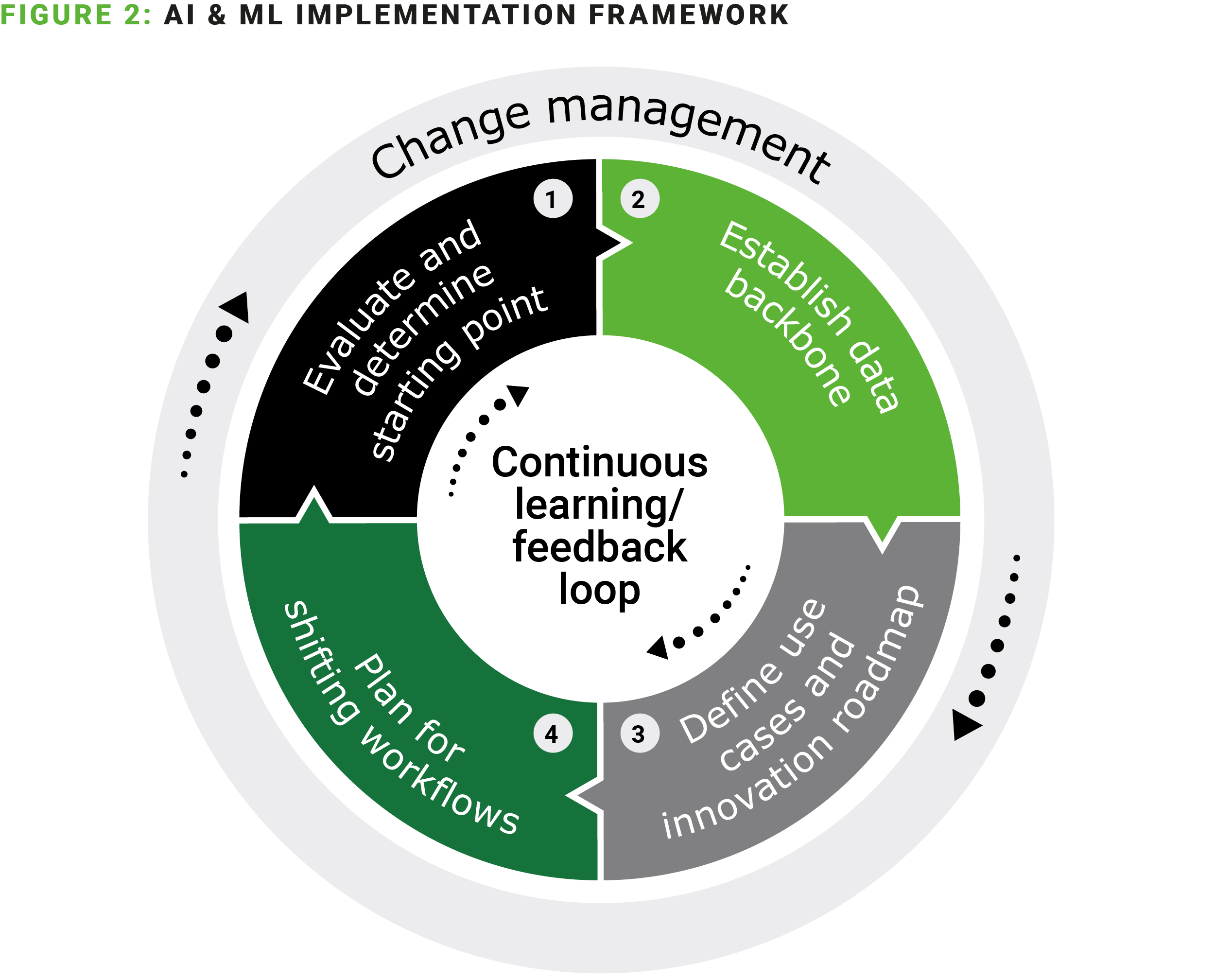
This article is an excerpt from our 2024 Media and Entertainment Predictions Report. You can view the full report here.